A lot of companies are considering implementing AI in their projects, but why would someone bother to do AI in the first place? In order to prevent disappointment, it’s crucial to understand what really works when it comes to AI.
- Insights hub
- /
- Approaching AI projects w...

Approaching AI projects with Robin Zondag
- DetailsAbout the talks
- TranscriptTranscript
- Resources2 Files
Approaching AI projects with Robin Zondag
Michał Grela
Relationship Manager at Future Processing
In this episode of IT Leadership Insights, Michał Grela talks with Robin Zondag on how to approach an AI project. A lot of companies are considering implementing AI in their projects, but why would someone bother to do AI in the first place? In order to prevent disappointment, it’s crucial to understand what really works when it comes to AI. In this talk, Michal Grela asks Robin Zondag how managing an AI project looks like, what skills should your team have to tackle it and how to align business, IT and data.
In this talk, Michal Grela asks Robin Zondag how managing an AI project looks like, what skills should your team have to tackle it and how to align business, IT and data.
Michal Grela: Hi. My name is Michal Grela, and I’d like to welcome you very warmly to yet another episode of IT Leadership Insights by Future Processing. My guest today is Robin Zondag. We’re going to talk about managing AI projects. AI as a technology is disrupting industry after industry, and managing a project management itself is a hard task enough, but AI makes it even way more complicated. Before we dig deep into the topic, Robin, would you be so kind and tell a few words about yourself, please?
Robin Zondag: Yeah, no problem, Michal. Thanks for having me in your podcast, video cast.
MG: Thank you.
RZ: Good to be here. My name is Robin Zondag. If you pronounce it in the Dutch way. Robin is what they do internationally. I’ve been working in IT industry for the last 20 years, spending most of my times at Atos in corporate, being responsible for digital transformation, AI and analytics and lastly, being responsible for the Google cloud AI business globally that Atos is doing. Since October 1st, I’ve changed course and I’m a corporate tyke turned entrepreneur, so I’m now running-
MG: You’re out of the nest.
RZ: It’s really good. I’ve learned enough after 20 years, I hope, and started my own company, Digital Sundai, focusing on doing digital business with AI technology, basically Google cloud stack.
MG: Thank you. Well, congrats on this brave move and becoming an entrepreneur yourself. As you’ve said, you have plenty of experience within product management and AI as a technology. I’m just curious to find out what’s your point of view, why would somebody bother to do AI in the first place?
RZ: Yeah. I think AI is a fantastic new technology that’s been around for a little while, but it’s now really being opening up to any organization, and it helps to make decisions better. Sometimes we don’t even realize, but there’s a lot of small decisions everybody is taking, and AI offers the opportunity sometimes. You have to think about where it’s useful to do this decision making automatically based on much more data that humans can’t oversee and leading to all sorts of new understanding like computers understanding voice, computers understanding, computers understanding images, facial recognition. Really opening up new ways of working, better ways of working which will service customers better and give the ability to companies to reduce cost. It’s also the difficulty, I think, with AI as we can apply it to so many different cases that we might not even know how to really use it. It’s really a big technology change that allows people to know how to apply it to be more competitive than organizations that do not apply it.
MG: I’m just also curious to understand, why now? If you’ve had these conversations on AI five or 10 years ago, everybody would be like, “Yeah, well, maybe later.” Whereas now, it’s the major topic of conferences, AI here, AI there, AI this, AI that. What’s changed? Why now?
RZ: Yeah. AI has an interesting history of being hyped up and-
MG: Yeah, definitely.
RZ: … people fearing that computers will take over and be much smarter than humans. I think first time probably in the ’60s, ’70s and ’80s again and then people got really disappointed on how smart computers really were. I think the last time was early ’90s when people thought, “Okay, now with the internet and all the data is becoming available, AI will take over.” Then, well, it didn’t.
MG: Nothing yet.
RZ: Not yet at that time. The big breakthrough, and I think what’s driving AI as a force right now is deep learning technology that allowed to continue to learn if you feed more data to models and make much more complex AI models that are closer to reality. It was technology invented somewhere around 2006 with Hinton and his team and many others contributing. That allowed … All of a sudden where in 2012 when it was about recognizing what’s on a picture, I think computers were failing 30% of the time, so performing way worse than any human would. A human error rate, if you show a picture and asked, what’s on the picture, is around 4%, 5%. With the deep learning technology, we saw that in three, four years so 2015, 2016, computers were actually more precise and more often right in recognizing what’s on a picture than humans. Now at an error rate of 3% for computers, whereas a human still … We haven’t really got the updates so we’re still at 5% wrong technically. That deep learning really got a breakthrough. Also, in terms of translation, in terms of speech, in terms of voice, now computers are getting on par, not with their global understanding of the world. That’s where humans beat computers anywhere, but on particular areas, particular focus areas, computers can really help humans to perform better.
MG: You said there’s hype. I recall once we’ve had this conversation when you said when there’s hype, there’s also always disappointment. I’m curious. Just thinking out loud with the … because there are some technologies definitely that people are waiting for them to get there, and they’ve definitely got there. I’m wondering if AI is just not another over-hyped thing.
RZ: It’s definitely in a hype phase, which means that anything that looks like data and some intelligence get labeled AI, which also means that people say, “Okay, I’ve got this business problem and I apply AI, and now the business problem is solved.”;
MG: Magic wand.
RZ: It’s like magic dust and all your problems are gone. That’s of course not true. We have to admit a little bit from our industry, it’s being fat … There’s a lot of over promising, and it’s really about finding the true value in the hype that will get us there. Because of the over promising, people will apply AI and still have some problems and think, “Oh my, God. They told me this would solve all my problems, and yet I still have problems, so I’m disappointed. I expected it to go faster and so on. With AI, as it requires, depending a bit on where you apply it, but it requires a lot of data, which a lot of organizations have a lot of data, but it’s usually not easily accessible. It’s a great effort to get that data, feed it to the machines. If the business case, and if you can’t scale up quickly enough, then you’re spending money on getting AI to work, which is let’s say more than you will do in an ultimately perfect world for AI-driven companies. That’s not available in most organizations. On the other hand, there are clearly a complete set and a growing set of areas and business cases where AI can really contribute.
MG: Right. Let’s let’s move to the other one. What’s the right fit for AI then where it works?
RZ: It’s all a matter … I think it’s a balancing act between effort and feasibility, the business value and how easy you can obtain that business value. For any organization that is having B2C conversations, a chatbot, which is basically driven by AI technology. You don’t need to build your own models. It’s been done by the industry already. It’s the natural language processing that’s behind it. That is bound to help you. We see an explosion of these chatbots being implemented. I think it’s growing more than 30% per year in terms of dollar amount, but it means almost any organization, how can I apply it? That’s a given one. It’s proven technology, and if you apply it right, you can really do it much better. Where it becomes a bit more of a research is when you are trying to use your own data and trying to get insights like the recommendations we see on the internet, next best offers and so on. Definitely a lot of success is in the industry, but sometimes also fairly because it’s not so easy to do a recommendation, which there’s a lot of data in B2C environments. It’s easier than B2B where there are larger deals but less deals and less information available. It also is a matter of feasibility and effort. How much effort is in your organization to get to the data, which for a lot of organizations quite a challenge. If I have a working model, how easily can I scale it and deploy it to get really the business value? Those are questions to be answered to be really precise in answering, okay, does AI for this case, is it a sensible solution? It’s a moving target as organizations are organizing themselves to be more AI friendly. There’s not so many corporates I know of that are not driving data quality programs, data access programs, data governance programs, training. They’re building their competence centers, training people on how to use AI if they’re marketeers or if they’re in finance and so on. With the growing maturity of organizations, you will see it’s going to become there are going to be more cases where AI is beneficial and achievable for organizations. At the same time, technology is progressing. A lot of providers, a lot of vendors are providing AutoML like AI, so you don’t need to be an AI expert, PhD and so on, but you can just be an ordinary software programmer that has of course some understanding of how to apply these models and still train and use these models because they’ve been pre-trained, and an API will help you and get you going. I think that’s also shifting the … Well, making it more accessible for many more organizations to do so. I’m pretty optimistic. I think I’ll still advise, really think about where you can apply it and where it makes sense right now. At the same time, this will grow for any organization in the coming years.
MG: I really like the bit you said that there’s some level of due diligence that must be made as a way of assessing whether we’re ready to do that, and it’s definitely not for everything and definitely not for everybody, but there are use cases where this technology can really contribute, and it’s just not another over-hyped thing. Say we’re a business where we can adopt this technology and there’s no off the shelf solution. We should build something ourselves. How should we even approach this project? Is it the same way as every other or some tweaks? How would you start something like that?
RZ: I think with AI, especially in this case, there’s no proven solution on the market already but you know there’s a lot of data, and insights in this data, for instance, predictive maintenance or forecasting your sales would really help in your logistical system and be more precise and cheaper and don’t have an empty shelf kind of thing. There’s clear business benefits. It starts with merging those two. It’s really a creative process in this case because it hasn’t been done before. Nobody really knows how good AI will be, and nobody really knows what would the AI-driven customer service of an airline look like, for instance just to take an example. It starts with an innovation phase where it’s really important to have the right subject matter expert from business in the room. It could be a design sprint, a week kind of thing, to define what needs to be done, to have AI experts in the room that understand as good as possible what is achievable and what’s not given the business doc and business process and the available data and the accessibility. To have IT guys in the room to really help understand in this particular organization, how easy would it be to get to the data. In creative process, which typically is a design thinking process where you need to facilitate it to be. You have to merge those two worlds together. It’s almost two worlds like the technology, AI. We can build this model, but what’s the value with your business process? Oh, if we tweak the business process a little bit and the value gets much more tremendously high, can we do this? Because it means 5,000 people need to work in a different way. We already have 25 programs running, so maybe not this year could be one of the conclusions. Yes, we can do it if we start with, let’s say, the first department and see how it really builds added value. It can be reasonably done, well with a reasonable amount of effort given the potential business value, and then you’ve got your case. It’s the creativity that, how do we change our business and organized with AI technology, and how difficult is it to build the right model and deploy and scale it across the company and the business process that is at hand? It’s always difficult to predict anything, so that’s why the world now is in agile mode and I think make good sense to start small, experiment trial lot and really get it right. Think a little bit. We don’t do something that everybody would say like, “Come on. This car is not going to fly. We know that this is not possible right now.” A little bit of thinking upfront and then a lot of experimenting, quick feedback loops. The multidisciplinary approach is it’s really combining technology and new ways that-
MG: I really like that bit about starting small and being agile. I believe that in today’s world of tech, you just can’t do it otherwise. You’ve said there’s IT, there’s business, there’s data on top of it, all over it. How would you align all these worlds that usually are siloed or not understanding each other? How would you make it thrive?
RZ: Yeah. I think to me, there are two kinds of answers to that. There’s the individual project where let’s say the organizational situation is a given, so they’re working in silos. There’s IT process which might still be a bit in the traditional side. We have a lot of change management steering committees. We have releases every four months, or somebody need to get into the release stuff. Let’s say an enterprise continuity focus. Let’s say that you have to operate and innovate in that environment, and maybe the business environment is also quite top down and innovation is not at the heart of the company, which for many traditional corporates is the case. In that case, it’s the team that needs to make it happen. The agile team needs to have those disciplines. It’s bound to be a bit more difficult than in let’s say a Silicon Valley-born, digital native startup where the data is easily available, where everybody works agile, and where there are releases a day on the website. Yes, the team will spend more effort to do the same thing in a traditional game, but it’s definitely possible if you make sure that you have the right support. This is quite classical stakeholders from business, from IT supporting the project, getting all of the competencies in the teams. Meaning, a scrum master that really knows his way around or her way around in the company and knows how to work agile, if the company maybe is not fully used to working in an agile mode, can really help. It’s more like an agile coach/scrum master than just a scrum master.
MG: Yeah, exactly.
RZ: You’ll need the subject matter expert who really understands, call it maybe the analytics translator, proper term right now, but basically somebody who really understands business. If it’s a customer service project, knows how this processes work, knows the do’s and don’ts and the absolutely don’ts, so it can really feed the team and focus on value. Plus, has the connections with the business teams to say, “Okay. Let’s try on this office to first apply it because this is where we have the people who are open-minded and where we have some chance of success.” That’s an important one. Then, you get to the data scientist, AI expert that needs to be able to model. You need to have expertise on board. That’s not an easy one because there’s many more AI projects right now than AI experts around. That is something that you need to spend effort to get the right person or team of persons to do it. Good news is it’s getting easier because of the, let’s say, AutoML offerings from the likes of Google and so on. Still, assuming that you have to build your own model, this is where you really need this person you might find outside or combined. I see a lot of projects where companies have hied data scientists, but they’re a bit young or a bit immature and work with external companies to … Okay, let’s do the work and then train at the same time the data scientist of the organization to do it the next time by himself. Plus, especially important in the first phase is building the models, getting the right predictions. The other part of the team is getting, and it’s not an easy job, is getting the data in the right format.
MG: Yeah.
RZ: Usually, 60, 70% of the time of these teams, especially in the beginning, are spent on just figuring out what data it is, getting the business approval to use it, and then getting the actual physical access to this data. There is work to get the right format so actually the models can learn from it. In the first phase when you try to, just on a small scale, trying to prove that your idea is visible from an AI and technology perspective, from a compliancy perspective as well, you don’t want to do all sorts of bias things or you violate privacy regulations. From a business value perspective, this is the team that can do it. If they got something working on a small scale saying, “Okay, we’ve got the model. We’ve got the small set of data that is underlying and proving our model, and we’ve got the business interaction that says, yes, if we do it this way, it helps. We now perform better. We can do the change that’s needed.” That’s the first, let’s say, MTP team. This is where a lot of companies go. Many companies achieve this. Where everybody is talking right now and say, “This is nice, but it’s not going to change my business results tremendously because I now have it on one office, but I have 3,000 offices around the world.”;
MG: Step one, yeah.
RZ: Yeah. Now we need to scale. That’s where let’s say some other skills need to be in this team.
MG: Completely different. Yeah.
RZ: Yes. This last modeling effort will have been done. There is a way to say, “Okay, now we need to change the business.” It’s a business change program. If anything, well, it doesn’t really matter. It’s an AI change, but it’s depending on how much the way working is changed. It’s easier or more complex. That’s just change management, training, communication, all that stuff on the business side that needs to be done. You need to have buy-in from people and understanding. That’s work. The other work is getting it accepted and connected with IT systems. Now you move into this four-month release kind of thing, and you have to align with all the standards that any corporate has deployed to make sure the continuity of the company is guaranteed. That is maybe you did some cloud, maybe you didn’t. There are APIs or interfaces or whatever, and getting into the right roadmaps, having the IT skills to build the data pipeline and continuously feeds the model and feeds back the results, so people actually working in Salesforce get the results, just an example, but working these enterprise systems get the results.
MG: Well, that was really insightful. Thank you. Thank you for that explanation. It’s definitely a hard task, a hard nut to crack, but I think it’s what sets successful organizations apart is that they just have the ability. They know how to create the right environment, whether it’s an AI project, whether it’s any other project. Either you have it or not.
RZ:Yeah.
MG:That’s what distinguishes leaders from the followers, I guess.
RZ:
I think so. It depends. Sometimes you can really make the small scale, so you don’t have to be very AI-friendly as a company and still have success. The other thing I see happen a lot is that let’s say organizations that have been successful for many years and successful in the industrial age are really transforming themselves to be much more digital-driven companies with way faster agility and therefore changing and breaking the silos anyway a little bit, changing their cultures. At the same time, opening up on the data, organizing the data much more with AI applications in mind, building up their competences to really changing their digital and AI-driven capabilities. There’s a lot of companies working hard. It’s quite a change, I think, from a successful company in the industrial age to a successful company in the digital age.
MG: Of course, yes.
RZ: There’s a lot of work, and it’s going to be step by step for most organizations, but you can see the leaders really moving fast, getting the right talent on board, getting to cloud native app development, which allows them to change much faster, getting an innovative culture in place. Probably the culture is the hardest thing to crack. Moving decision authority from top management to lowering your organization to speed up is a big change. If you’re on the stock market and have to perform after three months, you…
MG: Plenty of things that can be done. The cake is huge, but you have to eat the elephant bite after bite.
RZ: Absolutely.
MG: Yeah. Where are we, to shortly sum up this conversation? AI, there’s way more beyond the beehive when it comes to AI. If you are in the right place and have the right skills and the right attitude and the mindset in place, then as a company, you can definitely make the right use of it if you know how to do it.
RZ: Yes, definitely. I think on different levels, so you could apply it on specific areas and you don’t have to change all these things. You do it one day, you already profit. Introducing a chatbot does not require you to change the way of working at IT or the other parts of your organization. You can already profit. There’s a lot of point solutions that you can profit from if you want to make it. I think in the end, companies will want to make it an integral part of the way how you do business, how you organize yourself. A bit like with looking at Amazon and talking to them, and they use it as a standard part of any project and anything they do. It’s fully integrated, and it allows them to be faster, smarter in a lot of areas, HR or whether it’s logistics. If you want to go there, that is a big, big change for many companies, but you can already profit small. Time also depends on your industry, but you have to change so fast. If you’re in the media or travel industry, I think you don’t have a choice. Banks, you have to go fast. If you’re in, say, raw material selling, maybe you have a bit more time to make those changes.
MG: Thank you, Robin. It was very, very informative. I really enjoyed that one.
RZ: Michal, thank you.
MG: Thank you, our viewers and listeners for being with us in this yet another episode of IT Leadership Insights. If you liked it and if you found it useful, please don’t hesitate to share it and do drop us a line if you’d like to have a topic covered in one of the other episodes. Thank you. This is IT Leadership Insights by Future Processing with Robin? Thank you. Bye.
RZ: Bye.
INSIGHTS
Check similar insights
Contact
Get in touch
Have any question about specific material?
Let us know!
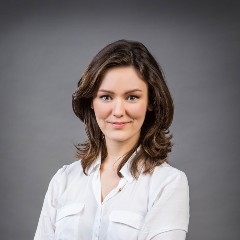
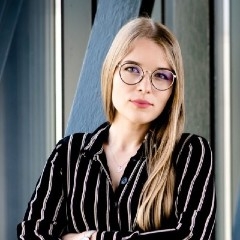
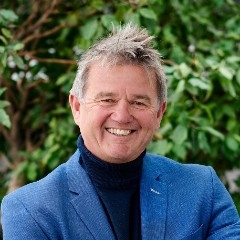